Topic 1: AI in CHE
(主題1: AI在化工的應用)
AI Soft Sensor Development (AI軟儀表開發)
Our laboratory focuses on AI Soft Sensor Development. We are developing time series models to predict quality parameters in chemical processes. These models analyze historical process data over time to forecast future quality values. This helps in areas like quality prediction in chemical manufacturing, allowing for proactive adjustments and improved product quality. (我們實驗室專注於AI軟儀表開發。我們正在開發時間序列模型,以預測化學製程中的品質參數。這些模型分析歷史製程數據,以預測未來的品質數值。這有助於化學製造中的品質預測等領域,從而實現前瞻性的調整並提高產品品質。)
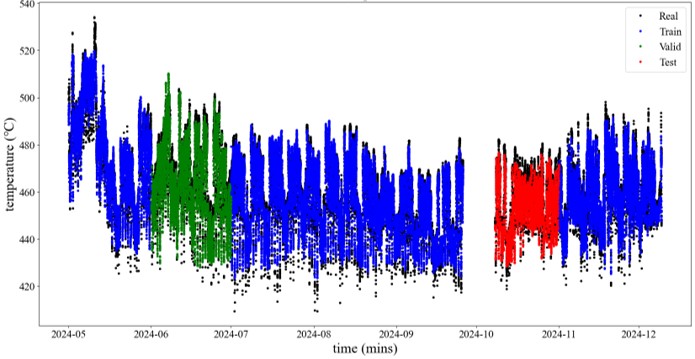
AI Controller (AI控制器)
Our laboratory focuses on developing AI Controllers for chemical processes, specifically using neural network model predictive control (NN-MPC) and reinforcement learning (RL). Our aim is to achieve objectives like net-zero carbon emissions. These advanced AI techniques analyze complex process dynamics and learn optimal control strategies to minimize energy consumption, reduce waste, and ultimately contribute to significant reductions in carbon footprint within the chemical industry. (我們實驗室專注於開發化學製程的AI控制器,特別是使用類神經網路模型預測控制 (NN-MPC) 和強化學習 (RL)。我們的目標是實現例如淨零碳排放等目標。這些先進的AI技術分析複雜的製程動態,並學習最佳的控制策略,以最小化能源消耗、減少廢棄物,並最終為化學工業內顯著減少碳足跡做出貢獻。)
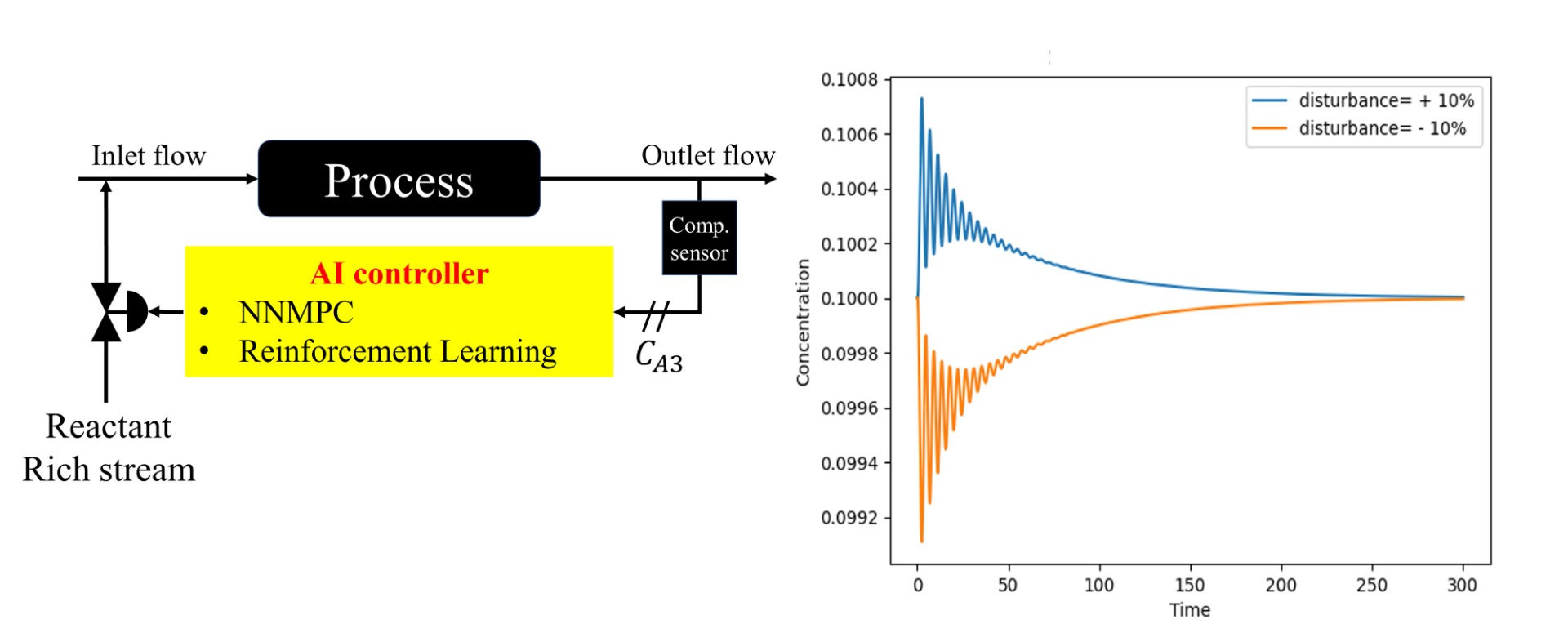
Machine Learning Force Fields: Transforming Classical Molecular Dynamics Simulations
(機器學習力場:轉變經典分子動力學)
Our laboratory is currently developing Machine Learning Force Field-based Molecular Simulation algorithms (MLFF). We are focused on creating these advanced models to enhance the accuracy and efficiency of classical molecular dynamics simulations, particularly for applications in chemical engineering. Our work involves leveraging neural networks and data-driven approaches to learn complex atomic interactions from high-fidelity quantum mechanical data. This allows us to simulate and predict the properties of polymer membranes with greater precision. (我們的實驗室目前正在開發機器學習力場 (MLFF)演算法。我們專注於創建這些先進的模型,以提高分子動力學模擬的準確性和效率,特別是在化學工程領域的應用。我們的工作涉及利用神經網路和數據驅動的方法,從高精度的量子力學數據中學習複雜的原子相互作用。這使我們能夠以更高的精確度模擬和預測高分子薄膜的特性。 )
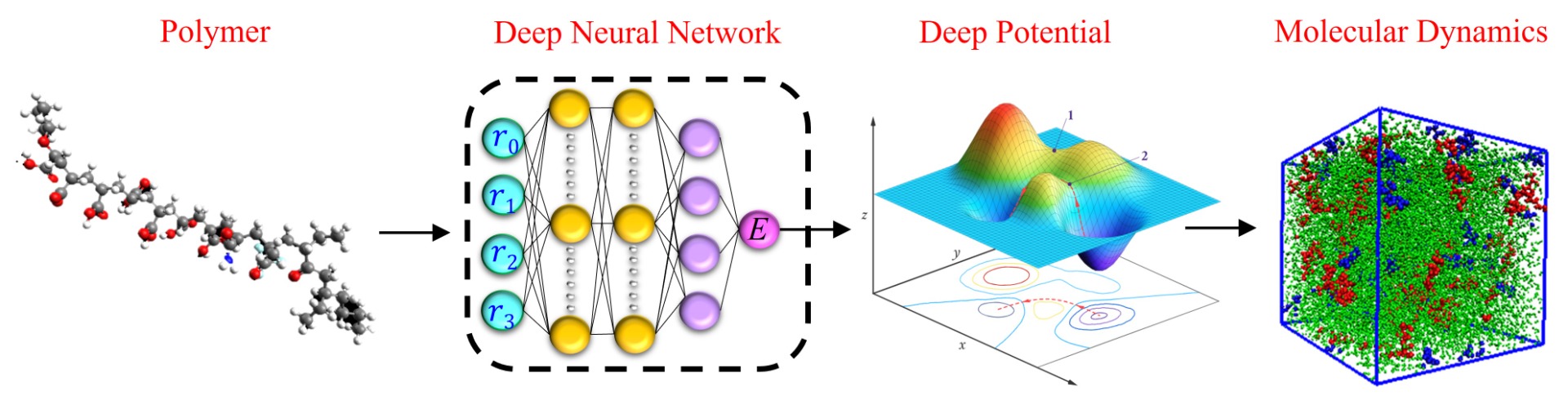
Topic 2: Polymer Membranes
(主題2: 高分子薄膜)
Experimental and Simulated Solid Polymer Lithium Batteries (實驗與模擬固態高分子鋰電池)
Our laboratory is actively developing solid-state lithium batteries. We are combining the experimental preparation of solid polymer electrolytes with machine learning force field (MLFF) molecular dynamics simulations to simulate, interpret, and predict the key properties of both the solid polymer electrolytes and the solid electrolyte interphase (SEI) with enhanced precision. This integrated approach aims to address the critical challenges of the SEI in next-generation batteries, ultimately accelerating the development of safer, longer-lasting, and higher-energy-density energy storage solutions. (我們實驗室目前正積極開發固態鋰電池,實驗製備固態高分子電解質結合機器學習力場 (MLFF) 分子動力學模擬,旨在以更高的精確度模擬解釋和預測關鍵的固態高分子電解質特性與材料開發,並解決下一代電池中固態電解質中間相 (SEI) 的關鍵挑戰,從而加速開發更安全、更長壽命和更高能量密度的儲能解決方案。)
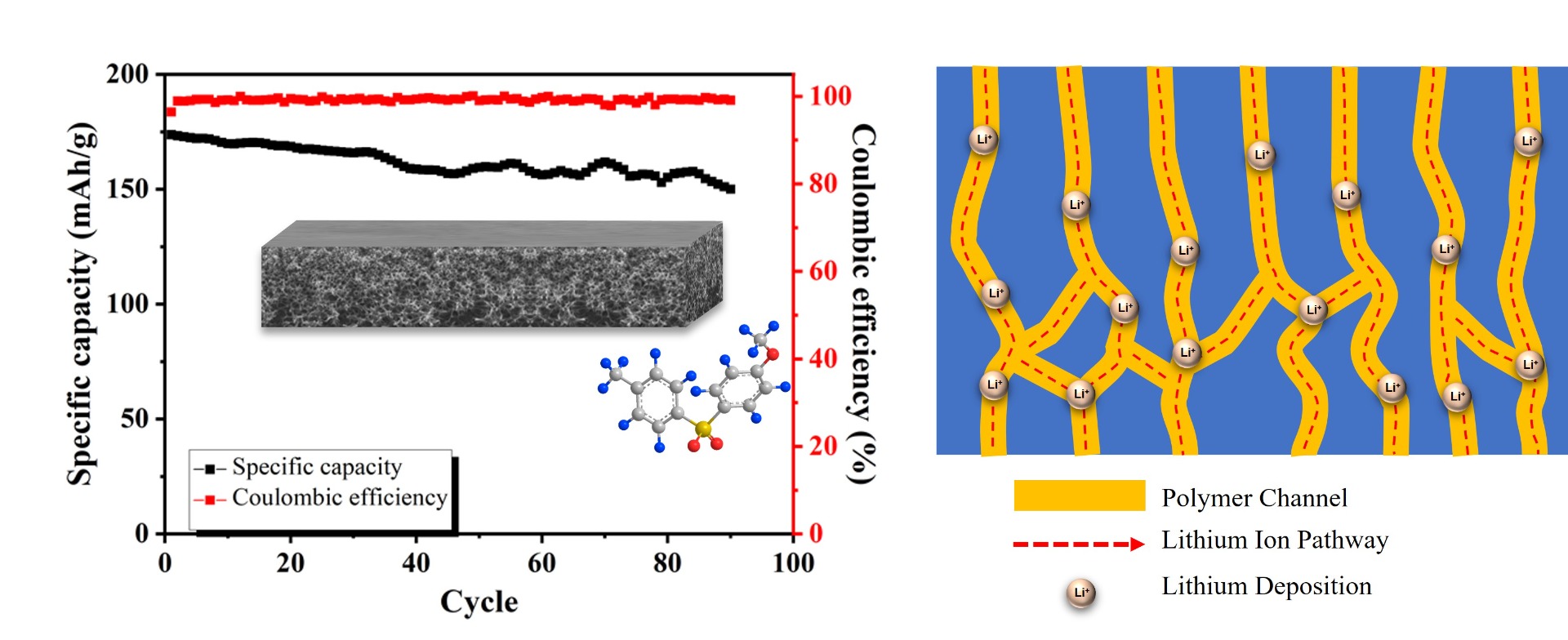
膜分離 (Membrane Separation)
Our laboratory has developed high-flux and high-resistance polymer membranes using non-solvent and vapor-induced phase separation methods, achieving superior performance compared to existing literature. Our work has applications in separation processes such as seawater desalination, wastewater treatment, and protein separation. (我們實驗室利用非溶劑與蒸汽誘導相分離方法開發出具有最高通量與阻隔率的高分子薄膜,應用於分離程序,像是海水淡化、廢水處理和蛋白質分離等。)
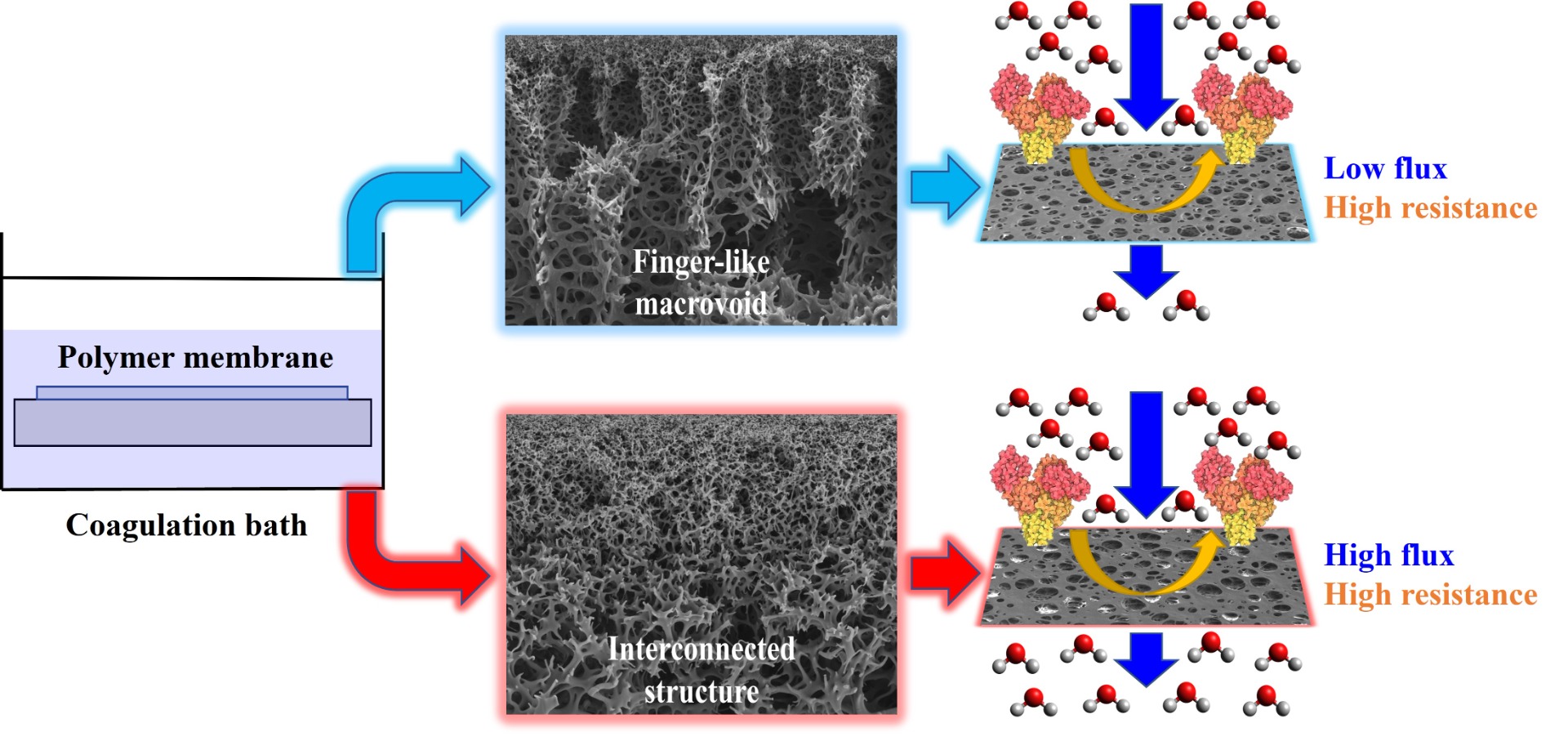